Imagine you’ve recently started an online fresh vegetable business. You have a catalog for fruits and vegetables explaining price and availability. Although most of the information is clearly mentioned on the website, you get hundreds of emails and phone calls regarding deliveries, discounts, and availability of your services in a particular location. Now, you could appoint someone for customer support and reply to these queries or simply — can implement a chatbot on your app and website that instantly answers such routine questions.
[Related: Conversational Chatbots for SMEs to continue business from home]
Chatbots for business are the need of the hour. The reasons are obvious. It is efficient, reduces workload, and responds to customer requests immediately. Nearly 1 in 4 customers have interacted with a brand via chatbots in the past 12 months, according to a Salesforce study published in late 2018.
As more and more customers are using e-commerce and digital medium for purchases, the incoming requests have also increased at the same rate. Companies need a larger workforce to handle customer support, failing which may lead to dangling customer satisfaction. Immediate query resolution also implies better customer experiences.
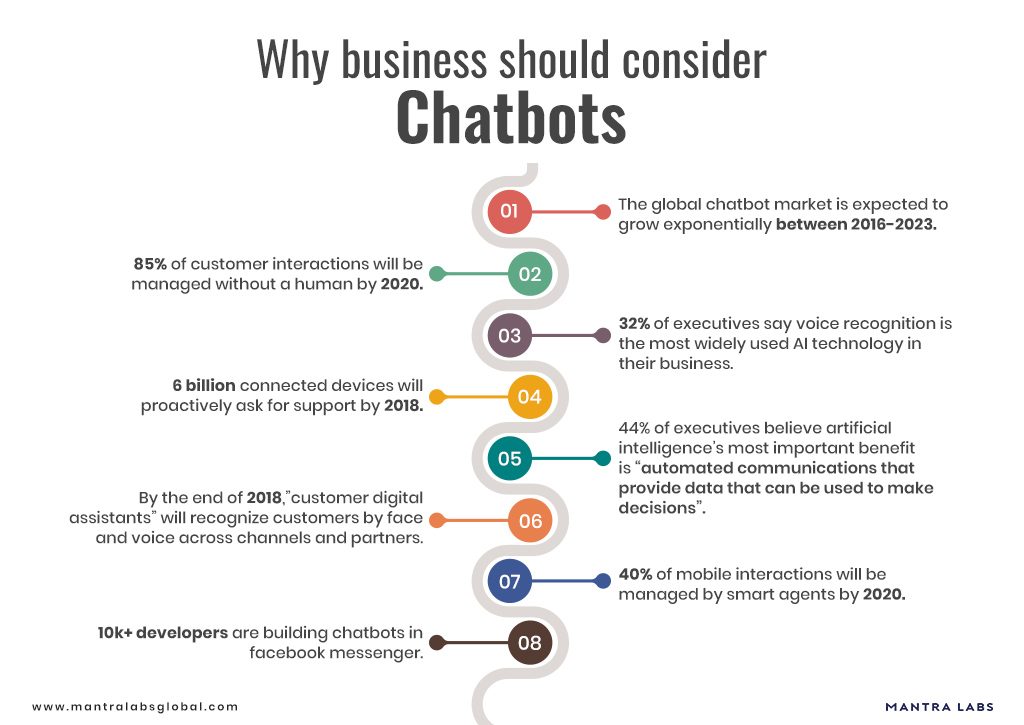
Chatbots for business: benefits at large
1. Humanized conversations
NLP-powered chatbots have the power to initiate and handle conversations with humans based on a set of predefined rules and upgrade its dictionary based on learning. Chatbots are a game-changer in terms of overall customer satisfaction pushing the market to reach 1.34 billion by 2024. As per the reports, smart chat agents will manage 40% of mobile interactions by 2020.
2. Easy to implement
A myth surrounding chatbots was doing rounds that it is expensive and exclusive to only fortune 500 companies. But, this is no more the case as it is predicted that by 2020, 85% of the chat interactions will be automated and will not need human intervention. In recent months, several new players like the virtual banker and progressive native chat have introduced schemes that help companies to set up chatbots instantly with reasonable investments. Also, 10K+ developers are building chatbots with the Facebook messenger.
3. People prefer self-serve interactions
Today, millennials represent 27% (2 billion) of the global population. This tech-savvy generation prefers immediate resolution to their concerns and instead of talking to the support, they’re happy about settlements over chats.
Making a customer happy is what all businesses need, and chatbots serve this purpose adequately. They are capable of resolving customer queries in just a few seconds, eliminating wait times and queues. It is a win-win situation for both the consumer and the provider as the customer gets instant replies and the provider saves on operational costs. By the end of 2018 automated customer agents will be able to recognize their customers through voice and face recognition.
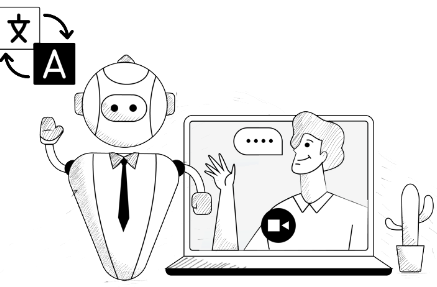
AI in Insurance will value at $36B by 2026. Chatbots will occupy 40% of overall deployment, predominantly within customer service roles.
DOWNLOAD REPORT
4. Fact-based decision making
All the conversations accomplished through chatbots are recorded and this contributes to the database for training future NLP models for more humanized conversations. Also, the data collected can help identify business bottlenecks and customer preferences towards specific products or services. All these, sum up to providing fact-based analytics for effective decision making.
5. Continuous innovations
Chatbots are here to stay. The innovations around chatbots are still in progress and time is not far when one will witness intelligent bots capable of resolving complicated issues on its own. We’ve already seen voice and vernacular chatbots in the market.
Big Techs are working on AI and machine learning to make smart chatbots that can offer much more than simple answers. If you haven’t thought about chatbots yet, then certainly you are missing on a significant business opportunity.
The significance of chatbots is already depicted in banking and marketing, and with time its influence will subsequently increase. Customers also expect chatbots and automated assistants from their business providers. They like to engage in live-chat as it helps them to get answers to their queries instantly. As of now, chatbots are only used for simple conversation. But, in the coming future, it will handle complex decision-making tasks. Any business that wants to evolve should consider chatbots and make it an integral part of their business.
We’re the makers of the world’s first insurance-specific chatbots. For further queries, please feel free to reach out to us at hello@mantralabsglobal.com.
Related Reads:
- Reimagining Medical Diagnosis with Chatbots
- 5 Ways HR Chatbots are Simplifying Hiring & Employee Engagement
- The three Vs of today’s chatbots: Voice, Vernacular and Video.
- Conversational Chatbots for SMEs to continue business from home
- Healthcare Chatbots: Innovative, Efficient, and Low-cost Care
- How chatbots are changing the digital Indian
Knowledge thats worth delivered in your inbox