It’s very human to skip tests. But, while developing enterprise apps, testing is something that should never be compromised. If you don’t test, there will be no way to find out the application performance and determine user experiences.
Testing is a must! You might already know that you should write tests for your code and UI, but you might not know — how? I’ll walk you through types of tests that developers usually perform on Swift programming language in order to help you deliver a supreme-quality app to your user.
Whether you’re building a new application or expanding the existing app, you might want to test it on the go. Testing in swift is as simple as building the app itself. (For your information, the Xcode also tests the application). All you need is test cases and an idea about where code usually goes wrong.
But first, it’s necessary to find out what to test.
Developing an App? What to Test?
Start with the basics. You must write mandatory tests if you plan to expand the application.
Tests usually cover the following issues.
- Core functionality: Model classes and methods and their interactions with the controller
- The most common UI workflows
- Boundary conditions
- Bug fixes
Let’s take a quick look at the types of testing while developing an app in Swift.
#1 Unit testing using Xcode
It is a process of creating small functionality-based tests for a particular unit of code, which will eventually ensure that all other units will pass the test.
The Test navigator provides the easiest way to work with tests; you’ll use it to create test targets and run tests against your app.
#2 UI Testing
UI testing is useful for testing interactions with the User interface. In UI testing, the developer needs to find the app’s UI objects through queries, synthesizing events. Tester has to then send the events to those objects. The API lets you examine the UI object’s properties and state which you can compare against the expected state.
#3 Performance Testing
A performance test uses a block of code that you want to evaluate. It is then run 10 times to collect the average execution time and the standard deviation for the runs. The average of these individual measurements (of the test run) are compared against the from a benchmark value to evaluate the success/failure of the project.
It’s very simple to write a performance test: You just place the code you want to measure into the closure of the measure().
Bonus – Code Coverage
The code coverage tool tells you about the parts of code that were actually executed during your tests. This way, you’ll know the parts of the app code that aren’t yet tested.
You can enable code coverage by editing the scheme’s Test action. Post this, check the Gather coverage for check box under the Options tab:
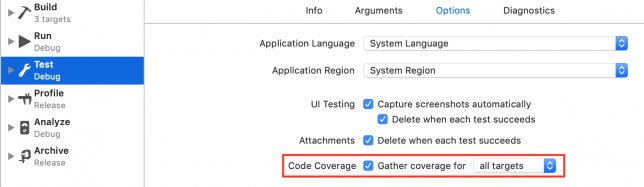
Now:
- Run all tests (Command-U)
- Open the Report navigator (Command-9)
- Select Coverage under the top item in that list (image below):
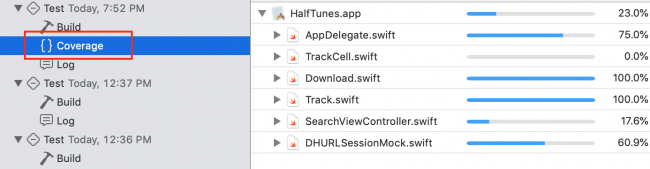
You can see the list of functions and closures in SearchViewController.swift by clicking the disclosure triangle:
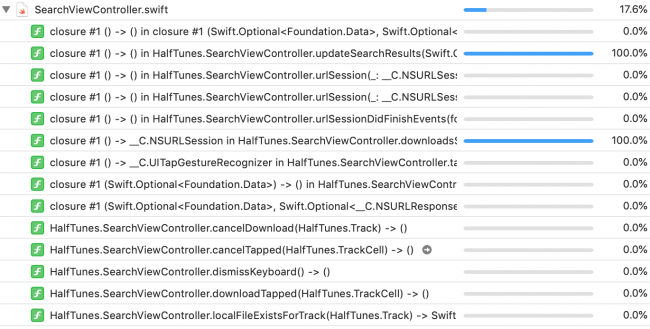
Scroll down to updateSearchResults(_:) to see that coverage is 87.9%.
Now:
Click the arrow button for this function to open the source file to the function. As you hover over the coverage annotations in the right sidebar, sections of code highlight green or red:
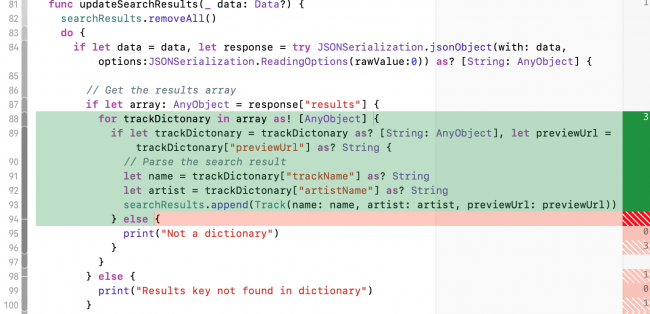
The coverage annotations show how many times a test hits each code section. Sections that weren’t called are highlighted in red. This implies — the for-loop ran 3 times, but nothing in the error paths were executed.
You can also increase the coverage of this function by duplicating abbaData.json, then edit it so it causes the different errors. For example, change “results” to “result” for a test that hits print(“Results key not found in dictionary”).
We help enterprises mitigate technical & business risk by securing vulnerable blind spots. Check out our testing services.
For your specific requirements, please feel free to drop us a word at hello@mantralabsglobal.com
About the author:
Anand Nanavaty is a Software Engineer with Mantra Labs. He has been deeply involved in mobile app development for the company’s B2B clients. Apart from coding, testing and experimenting with different application development frameworks, Anand loves travelling, trekking, mountaineering, sports (especially cricket), watching movies and sometimes making short films.
Further reading:
- Mobile app development trends
- Swift programming language: an overview
- React native framework: an in-depth study
- WWDC20: 6 Latest Additions in SwiftUI for iOS Developers
- 5 Key Takeaways for iOS Developers from WWDC 2020
For in-depth understanding of testing in Swift, you can refer to — Writing Test Classes and Methods
Knowledge thats worth delivered in your inbox