The healthcare industry is getting a comprehensive digital facelift. Digital Health Systems (DHS) that use new digital technologies like artificial intelligence & robotics are delivering smarter healthcare services and better health outcomes to the masses. Health organizations are increasingly relying on them to improve care coordination, chronic disease management and the overall patient experience. These health systems are also alleviating repetitive administrative tasks from the roles of healthcare professionals, allowing them more time to practice actual healthcare.
The Modern Medical Enterprise draws on digital-enabled technologies such as telemedicine, AR/VR and remote-monitoring wearables to diagnose diseases and promote self-care. These applications rely on high-volume processing of patient data on a frequent basis. Healthcare organizations also need to share/receive this information securely over a distributed network. However, sharing patient information remains a challenge, while the inability to access these records in a time-sensitive manner can affect the time-to-treatment for patients.
Deploying digital health systems that are both compliant to regulatory standards and functionally stable for a large number of concurrent users requires significant manned effort. Moreover, QA teams comprised of manual testers may end up working on repetitive manual test case scenarios that can lead to challenges in scaling or rolling out new features.
How can the modern healthcare enterprise keep pace with issues posed by the safe deployment of their digital health systems? Automated Testing is a hallmark process of any digital transformation project. It gives enterprises the ability to shorten their release cycles and meet their business needs without affecting productivity or operations across the healthcare value chain. Test Automation also allows medical enterprises to run repeatable and extensible test cases against real-world scenarios.
Test Automation Use Case
The growth of DevOps and the rise of mobile-first applications are responsible for driving the growth of the test automation market globally. Today, enterprises are able to go faster-to-market owing to the technological advancements in quality assurance & testing.
For instance, in the case of a large US-based teleradiology firm that offers enterprise Imaging Solutions for improving patient care — a stable and reliable system mandated custom-built test automation frameworks. The medical technology company provides fast & secure access to diagnostic quality images using any web enabled device. To achieve this, they have built a cloud-based image sharing platform that allows digital image streaming, diagnostic & clinical viewing, and archiving for healthcare organizations.
Medical Image sharing among healthcare organizations is altogether brimming with security risks, and requires a complex network of systems to facilitate its smooth functioning.
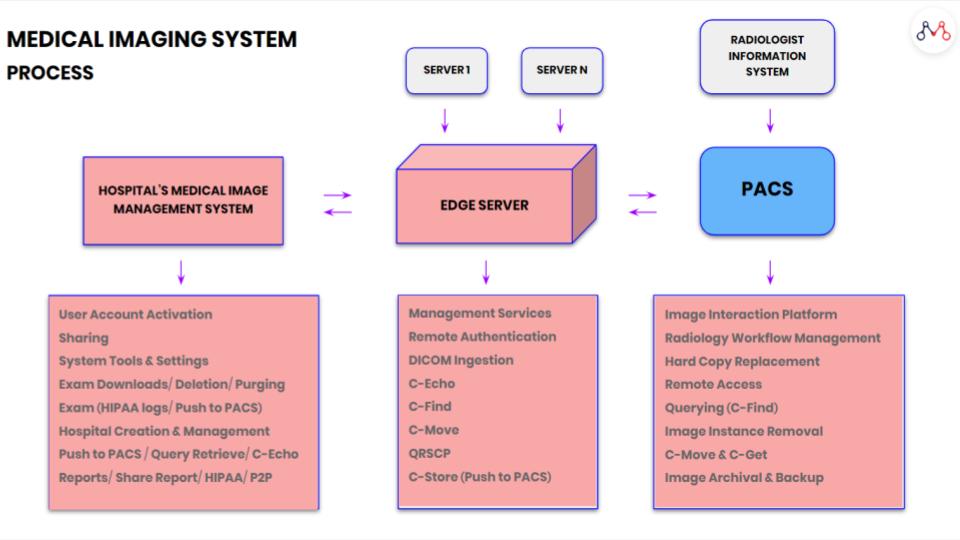
Also read – How are Medical Images shared among Healthcare Enterprises?
In order to fulfil their business objectives, Mantra Labs identified key challenges for their testing requirements, namely —
1. Scalability
The platform must be able to support a high number of concurrent users.
2. Fail-over Control
The platform should behave functionally correct under very high loads with stable fail-over capability.
3. Efficiency & Reliability
The platform must scale rapidly when supporting a large user base & multiple formats with minimal page navigation response time.
Several testing components were deployed along with test automation techniques to address the full range of QA issues, including: functional testing, integration testing, GUI testing, and regression testing.
Mantra Labs created a federated architecture to ensure near-perfect scaling, and true load & data isolation between different tenant organizations. The federated architecture consists of a number of deployments and a central set of components that stores global information like lists of organizations & users, and provides a centralized messaging service.
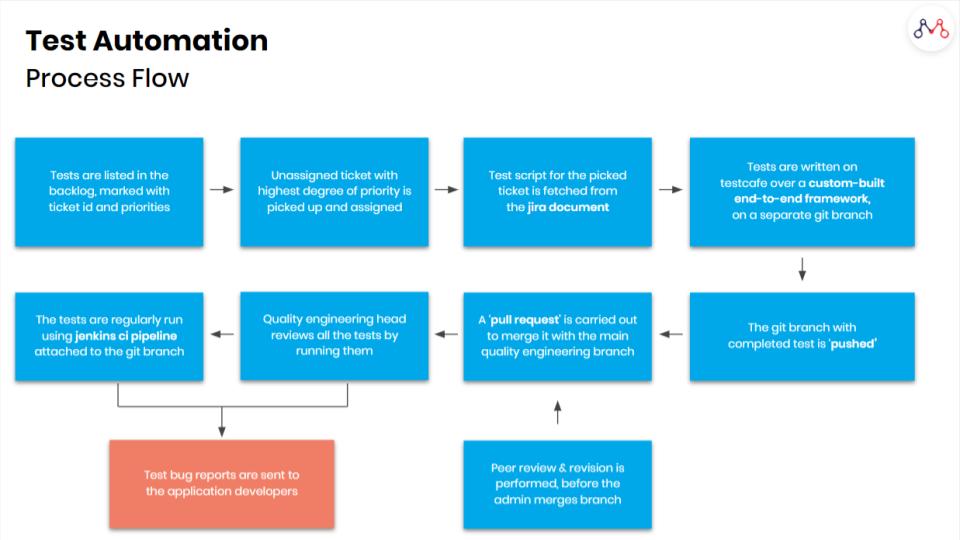
Test Automation Improves Accuracy & Test Coverage
The entire cycle of bug detection in the UI, API and Server Loads involves several weeks of regression manual efforts. By automating tests, techniques like Stochastic Tests can be applied to detect bugs and reduce the overall cycle time.
Through Mantra Labs deep medical domain expertise, in-depth testing practices, intuitive suggestions for platform scaling and successful test automation efforts — significant business objectives were realised over the course for the client. Mantra was able to achieve over 60% reduction in cycle time, and about 65 per cent improvement in bug detection capability before the release cycle.
Nearly 35% of Executive Management objectives revolve around implementing quality checks early in the product life cycle, which can be achieved through test automation. For further queries and details about automated testing, please feel free to reach us at hello@mantralabsglobal.com
Related:
- Test Automation Services
- Regression Testing in Agile: A Complete Guide for Enterprises
- Medical Image Management: DICOM Images Sharing Process
- Basics of load testing in Enterprise Applications using J-Meter
- [Part 1] Web Application Security Testing: Top 10 Risks & Solutions
- [Part 2] Web Application Security Testing: Top 10 Risks & Solutions
Knowledge thats worth delivered in your inbox