The insurance industry is changing and experts predict — nearly one-third of existing insurance models will disappear within this decade. The fierce competition, new opportunities with technologies like AI, and on top of that millennials’ changing preferences sum up to the call for more flexible and consumer-facing business models. Here are four new business models to set the insurance archetype.
Source: The Deloitte Global Millennial Survey 2019
Social Good & Transparency as a Business Model
Currently, AI is being used to strengthen the capabilities and knowledge of insurers and not consumers, creating information asymmetry. But, the question is — for how long will the consumers accept being a victim of ignorance.
A possible solution to this situation is bringing information transparency. It’s not like traditional insurers don’t share policy information with their customers. They do. However, lengthy policy documents and customers’ reliance on agents for information shadows the actual coverage, terms, etc. In a way, the information that customers receive becomes dependent on the agents’ knowledge and intentions.
Translating policy, terms and conditions documents into consumable bits of information with a clear distinction between what’s covered and what’s not will help in achieving transparency between insurers and customers.
For instance, Lemonade — the American Insurtech for renters and home insurance, disrupted the industry lately with their instant and transparent end-to-end insurance process. Their consumers are better aware of coverage and claims thanks to simplicity in the user experience.
Moreover, Lemonade donates the unclaimed premiums to social causes their consumers care about. From its inception in 2015 to date, Lemonade has sold over 1.2 million policies, in complete transparency and all through their AI bot — Maya!
Nearly 46% of millennials are willing to make a positive impact on the society/community. Lemonade has partnered with 92 charities and has donated $8,46,849 from unclaimed premiums. Hence, the answer.
Similarly, Swedish InsurTech Hedvig has successfully deployed it’s “nice insurance” services, giving back 80% of the unclaimed premiums to charities chosen by the customers.
More insights on — millennials and their expectations from insurance ‘beyond’ convenience.
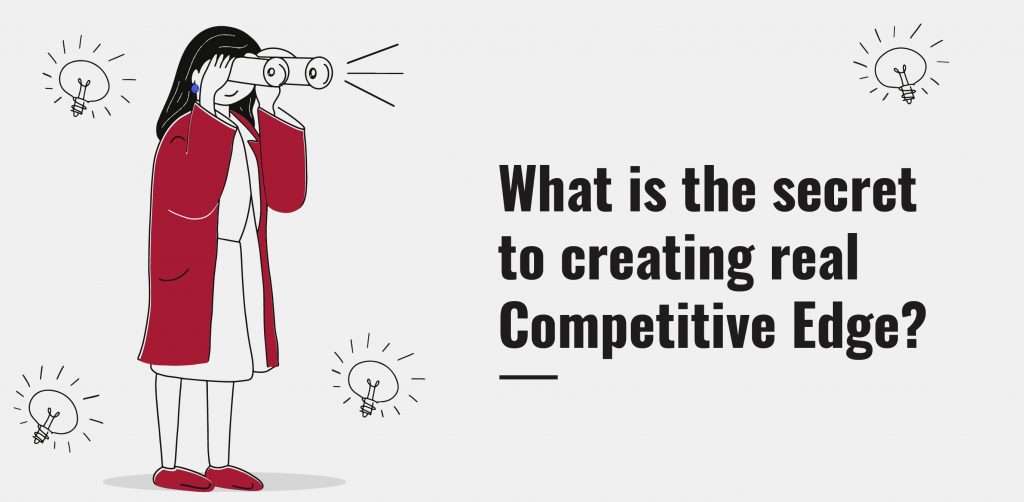
Join our Webinar — AI for Data-driven Insurers: Challenges, Opportunities & the Way Forward hosted by our CEO, Parag Sharma as he addresses Insurance business leaders and decision-makers on April 14, 2020.
B2B2C or API-based Model
When user acquisition is the top priority, B2B2C or API-based model comes into action. Also known as an open-source platform solution, this business model connects people and processes with technology infrastructure and assets to manage user interactions.
In the API-based model, apart from traditional distribution channels, 3rd party apps also become a medium for customers to buy/access insurance policies. Automation plays a key role in this insurance model. Here, any other customer-centric digital application can install the API without manual/human intervention.
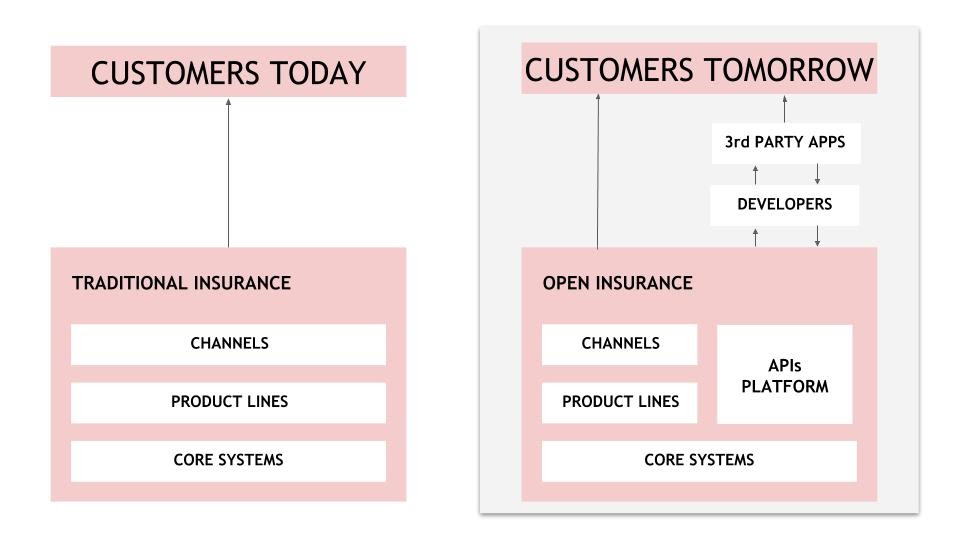
For example, in January 2018, Allianz announced that it will offer parts of its Allianz Business System (ABS) to other insurance companies for free. Interested organizations can simply install the API (Application Programming Interface, which is nothing but a chunk of software that connects two different apps) and start selling Allianz policies to their customers.
Lemonade — after disrupting the insurance space through transparency, has now stepped into this model. In October 2017, the company launched its public API, allowing anyone to distribute Lemonade’s policies through their websites or apps.
“It takes years to pull together the licenses, capital, and technology needed to offer insurance instantly through an app, which is why it’s almost nonexistent. Today’s API launch changes that. Anyone with a slight familiarity with coding can now include these capabilities in their app, in a matter of hours.”
Shai Wininger, Co-founder, President & COO, Lemonade
P2P Insurance
Unclaimed premiums also contribute to conflicts between insurers and policyholders. What if a customer is not interested in donating to charity, unlike mentioned in the above case?
Peer-to-Peer (P2P) insurance is perhaps an answer to eliminate premium settlement conflicts. It is also an emerging business model to access insurance coverage at lower costs than most of the traditional insurances.
This insurance model pools the individuals who share at least one relation — friends, family, or interest (community/clubs) and it serves two-fold benefits-
- Every member knows other members, funds available, and claims initiated/processed. Therefore, irrespective of the information shared by the insurer, there’s a transparent collaboration among peers.
- Since the members know each other socially, there’s a negligible chance of fraudulent claims. For instance, in the US alone, insurance frauds amount to nearly $80 billion/year.
Also read – how behavioral psychology is fixing modern insurance claims
The notion of financial protection for the community has been prevalent in our societies since the 1600s. In the middle ages, the tradesmen followed the guild system (an association of craftsmen and merchants), where participants paid fees as a kind of insurance safety net. Though, the successful conceptualization of P2P insurance in the modern business models dates back to 2010 with German InsurTech — Friensurance. However, the P2P insurance model has credited the success to many more InsurTechs like Guevara, Axieme, TongJuBao (P2Pprotect), and PeerCover.
Microinsurance
The greatest limiting factor for the success of microinsurance is distribution. For example, in the US, 18% of the premium represents the distribution cost, set aside marketing and advertising costs. Availability isn’t the issue for microinsurance.
The new business model for microinsurance focuses on outreaching and distributing policies at scale. Workflow automation solutions like document processing, automated customer query resolution, etc. make microinsurance models more effective.
- Aggregator model: Instead of traditional agents, retailers, utility or mobile network operators, etc. can be intermediaries for the distribution of microinsurance policies. They provide access to a very large consumer base and even more with free and freemium coverages. For example, Check24, a European aggregator together with HDI insurance developed AurumPROTECT that is available exclusively through aggregators channels.
- Harnessing proxy insurance sales force: Banks have been the ideal partners to distribute microinsurance policies at scale for ages. But, for short-term policies, this is a good time to utilize the agents of other products to offer insurance as an ancillary product. For example, Ola — an Indian cab aggregator provides a number of travel-related microinsurance underwritten by Acko General Insurance.
The Bottom Line
The effectiveness of each of these models drills down to the smart use of technology in their implementations. Moreover, most of these business models are automated, thus, eliminating additional human resources for implementations. For instance, in India, an agent can charge up to 20% of the premium amount as fees, which can reduce significantly if the distribution is automated. Investment in technology for automating operations is also worth it because it makes customer outreach simpler and faster.
Also, read – 5 Front-office operations in Insurance you can automate with AI.
Knowledge thats worth delivered in your inbox