Originally published on medium.com
If you’ve ever been in the position of having to file an insurance claim, you would agree that it isn’t the most pleasant experience that you’ve likely ever encountered.
In fact, according to J.D. Power’s 2018 Insurance Customer Satisfaction Study, managing time expectations is the key driver of satisfaction — meaning, a prompt claim settlement is still the best advertisable punch line for insurance firms. Time-to-settle satisfaction ratings were found to be 1.9 points lower even when the time frame was relatively short and insurers still missed customer timing expectations.
So what should an established insurance company do, to be at par with the customer’s desires of modern service standards? The question becomes even more pertinent when the insurance sector is still lagging behind consumer internet giants like Amazon, Uber who are creating newer levels of customer expectation. Lemonade, MetroMile and others are already taking significant market share away from traditional insurance carriers by facilitating experiences that were previously unheard of in the insurance trade.
Today, Lemonade contends that with AI, it has settled a claim in just 3 seconds! While a new era of claims settlement benchmarks are being set with AI, the industry is shifting their attitude towards embracing the real potential of intelligent technologies that can shave-off valuable time and money from the firm’s bottom-line.
How AI integrates across the Insurance Claims Life Cycle
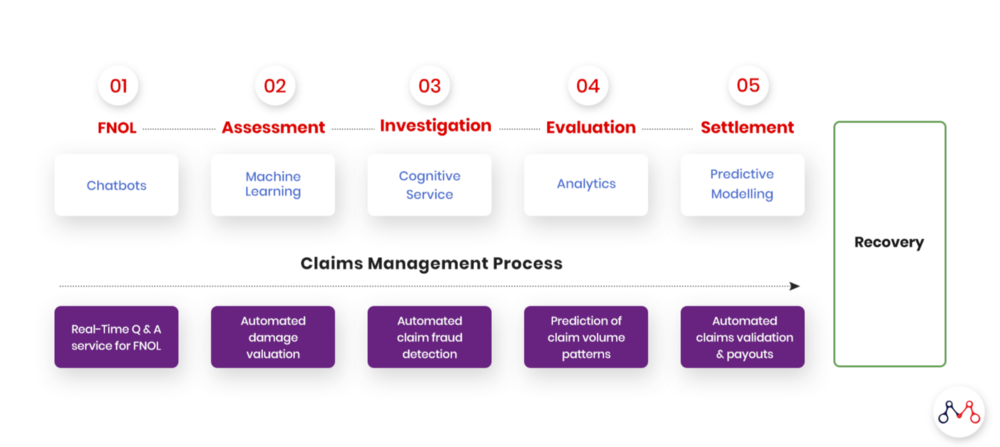
For this entire process to materialize — from the customer filling out the claim information online, to receiving the amount in a bank account within a short amount of time, and have the entire process be completely automated without any interference, bias, or the whims of human prejudice.
How does this come about? How does a system understand large volumes of information that requires subjective, human-like interpretation?
The answer lies within the cognitive abilities of AI systems.
For some insurers the thought that readily comes to mind is — Surely, it must be quite difficult to achieve this in real-world scenarios. Well, the answer is — NO, it isn’t!
Indeed, there are numerous examples of real-world cases that have already been implemented or are presently in use. To understand how these systems work, we need to break down the entire process into multiple steps, and see how each step is using AI and then passing over the control to the next step for further processing.
How It Works
For the AI-enabled health insurance claims cycle, there are a few distinct steps in the entire process.
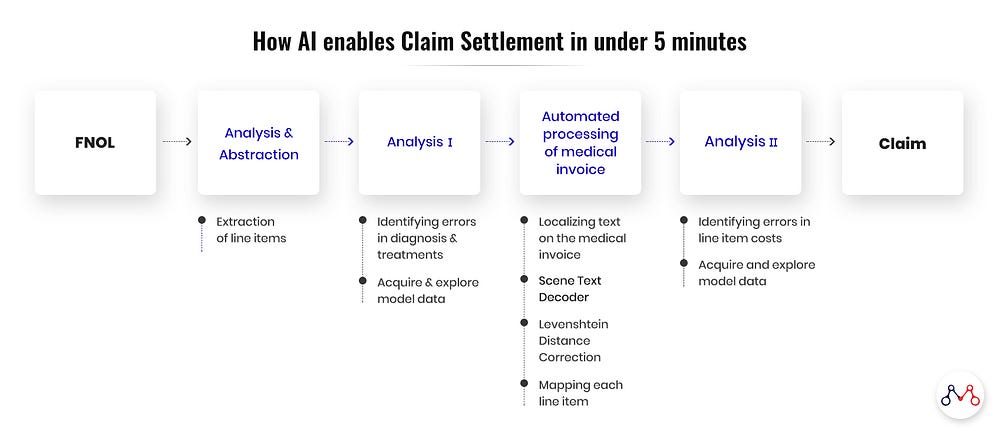
Analysis and abstraction
The following information is first extracted from medical documents (diagnosis reports, admission & discharge summaries etc.)
- Cause, manifestation, location, severity, encounter, and type of injury or disease — along with & related ICD Codes for injury or disease in textual format.
- CPT Codes — procedures or service performed on a patient, are also extracted.
There are in essence two different systems. The first one (described above) processes the information that is presented to it, while the other looks from the angle of genuineness of the information. The latter is the fraud detection system (Fraud, Abuse & Wastage Analyzer) that goes into critical examination of claim documents from the fraud, abuse and wastage perspective.
Fraud, Abuse & Wastage Analyzer
Insurance companies audit about 10% of their total claims. Out of which around 4–5% are found to be illegitimate. But the problem is that the results of these audit findings are available much after the claim has been settled, following which recovering back the money already paid for unsustainable claims is not that easy.
This means that companies are losing big sums on fraudulent claims. But is there a way by which insurers can sniff out fraud in real time while the claim is under processing?
With Cognitive AI technologies available today, this is achievable. All you need is a system that analyses hundreds and thousands of combinations of symptoms, diagnoses and comes up with possible suggested treatments. The suggestions are based on the learnings from past instances of cases that has been exposed to the AI system.
The suggested treatments’ tentative cost — based on the location, hospital, etc., is compared with the actual cost of the treatment. If the difference suggests an anomaly, then the case is flagged for review.
Automated processing of medical invoices
Now if your Fraud Analyzer finds no problem with a claim, how can you expedite its processing? Processing requires gathering information from all medical invoices, categorizing them into benefit buckets, and then finalizing the amount allowed under each head. Advanced systems can automate this entire process, ruling out manual intervention in most of these cases.
Recent AI systems have the capability of extracting line items from a scanned medical invoice image. This is achieved through a multistep process, outlined below.
- Localizing text on the medical invoice. This gives the bounding boxes around all texts.
- Running all localized boxes against a Scene Text Decoder trained using a LSTM and a Sequence Neural network.
- Applying Levenshtein Distance Correction for better accuracy.
- Mapping each line item against an insurer specific category.
Each line item is iterated over and looked up against the policy limits to get its upper limit. Each line item amount is aggregated to finally get the final settlement amount.
If the final settlement amount is within the limits set for straight through processing and no flags are raised by the Fraud, Abuse & Wastage Analyzer, then the claim is sent to billing for processing.
Moving Ahead With AI Enabled Claims
Today, AI transforms the insurance claims cycle with greater accuracy, speed and productivity, at a fraction of the cost (in the long run) — while delivering enhanced decision making capabilities and a superior experience in customer service. While, in the past, these innovations were overlooked and undervalued for the impact they produced — the insurers of today need to identify the proper use cases that match their organization’s needs and the significant value they can deliver to the customers of tomorrow. The cardinal rule is to — start small through feasible pilots, that will first bring lost dividends back into the organization.
Knowledge thats worth delivered in your inbox