According to Oracle’s Executive survey, 80% of leading consumer-facing businesses have already used or are planning to use chatbots by 2020. Chatbots are scalable and cost almost nothing in operation as compared to their human counterparts. But, how practical is chatbot adoption for your business? Let’s see.
5 Key Success Metrics for Chatbots
Different industries can utilize chatbots to serve different purposes. Accordingly, the parameters to measure ROI may vary. For instance, marketers may consider lead generation as a criterion while the sales department takes conversions from chatbots into account. But, of course, the decision to opt for chatbots depends on specific quantifiable measures — to solve specific customer support processes.
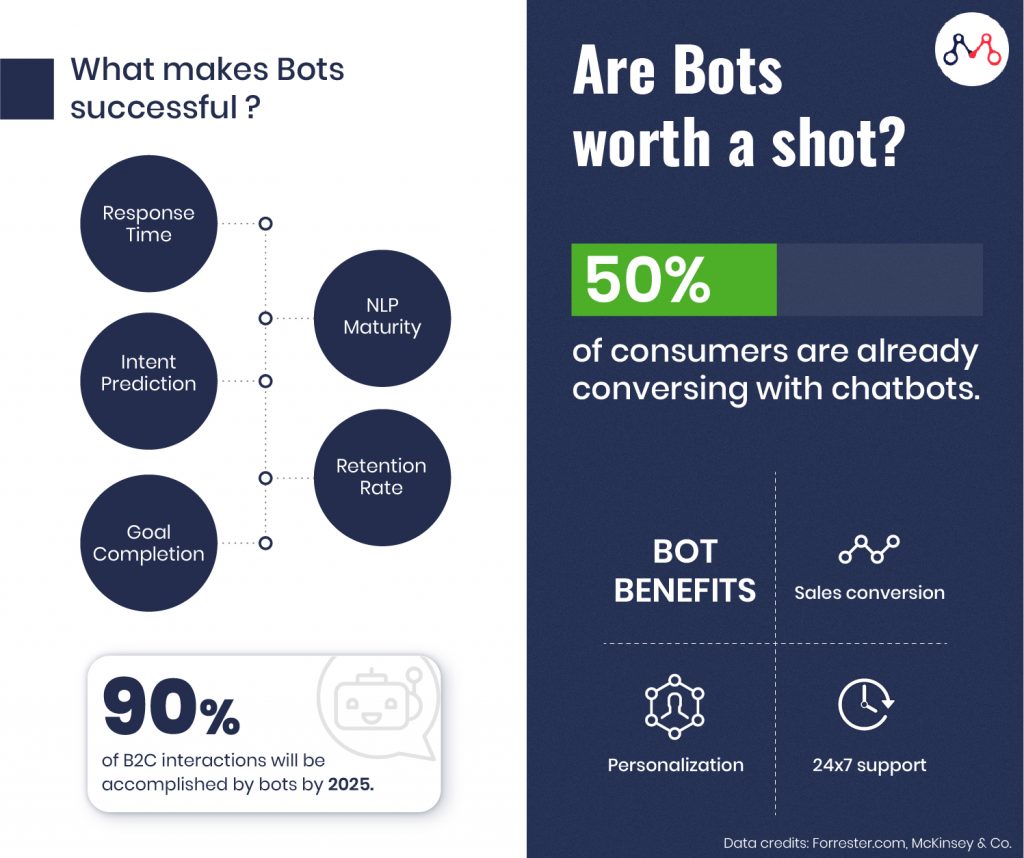
#1 NLP Maturity
It is the average maturity level of Natural Language Processing capability of bots, measured by the way bot interacts. Initiating conversations with customers is a key focus area among organizations these days. To achieve this, bots have to be well trained in industry-specific jargon.
For instance, if a retail customer has a question about a brand’s return policy, the bot should be able to meaningfully understand the user’s query and provide relevant information as it relates to that specific question, as opposed to an information dump or worse yet failing to understand the query itself. If a bot is unable to process the user input, it contributes to ‘miss-messages’. Such instances occur when the user inputs query in a regional or idiomatic language.
#2 Response Time
It is the average time taken for the chatbot to respond to customer queries, based on the total number of messages sent by a chatbot during an interaction. Typically this can average around 5-6 seconds. However, research indicates that users will leave a site if key elements take more than 3 seconds to load.
#3 Intent Prediction
It is the ability of the bot to anticipate what a customer wants in real-time. To achieve this, the bot must be paired with multiple sources of data and AI capable — in order to combine user behaviour, transactions, and profile details. Using this, the bot can determine intent based on both aggregated interactions for known and unknown users, and personalized data pulled from back-end systems.
#4 Retention Rate
It defines the number of users who willingly return to using the chatbot to address their issues. The retention rate varies according to industries. However, the clear formula for increasing user retention is to equip chatbots with the ability to understand user queries and empathically respond to them. This metric is directly correlated with the ability to personalize sales and/or customer service greetings, in 1:1 messaging.
#5 Goal Completion and Fall-back Rate
The number of times a chatbot can resolve the query, manage ticket, generates leads, or results in conversion determines its goal completion rate. However, like humans, bots, at times, might not be handle queries on their own. Such instances account for the fall-back rate of the bots.
Here’s an insightful read on why businesses should consider chatbots.
Successful Chatbot Adoption Across Businesses
Providing 24×7 support is not impossible for any organization. But, the labour cost associated is high, which makes chatbots a viable solution for instant customer support. IBM reports that globally businesses spend over $1.3 trillion/year to handle roughly 265 billion customer calls.
The following are examples of chatbots adoption for cost savings.
#Messenger Marketing Bot
ManyChat provides bot platform on Facebook Messenger for marketing, e-commerce, and support. DigitalMarketer incorporated ManyChat’s bot for messenger marketing and have reported very high returns on their ad spend (nearly 500% ROI).
#Insurance Chatbot
Religare has incorporated chatbot on its website and WhatsApp to handle customer queries. It has resulted in 10 times more customer interaction and 5 times more sales conversion.
Here are more insurance chatbot use cases.
#B2C Chatbot Offering Personalization
1-800-Flowers is using IBM Watson’s Gwyn smart virtual shopping assistant. It interacts with customers to understand their gift preferences and accordingly help them select a personalized gift for their loved ones. More than 70% of 1-800-Flowers customers are happily ordering through Gwyn bot.
Here’s a sample Chatbot ROI calculation from a financial perspective.
The Future of Chatbots
CNBC reports, currently businesses are saving $20 million per year globally through chatbot adoption. By 2022, chatbots can cut operational costs by more than $8 billion per year. Also, researchers predict that by 2025, bots will accomplish about 90% of the B2C interactions. Looking at the reduction in cost and ease of operation, investing in chatbots is worth it.
We specialize in building NLP and AI-powered chatbots for enterprises. Drop us a line at hello@mantralabsglobal.com to know more.
Knowledge thats worth delivered in your inbox